Stereoscope applied to left ventricule data#
Developed by Carlos Talavera-López Ph.D, WSI, edited by Romain Lopez
Version: 210301
In this notebook, we present the workflow to run Stereoscope within the scvi-tools codebase. We map the adult heart cell atlas data from Litviňuková et al (2020). This experiment takes around one hour to run on Colab.
You can access the raw count matrices as ‘anndata’ objects at www.heartcellatlas.org.
Note
Running the following cell will install tutorial dependencies on Google Colab only. It will have no effect on environments other than Google Colab.
!pip install --quiet scvi-colab
from scvi_colab import install
install()
WARNING: Running pip as the 'root' user can result in broken permissions and conflicting behaviour with the system package manager. It is recommended to use a virtual environment instead: https://pip.pypa.io/warnings/venv
import os
import tempfile
import matplotlib.pyplot as plt
import numpy as np
import scanpy as sc
import scvi
import seaborn as sns
import torch
from scvi.external import RNAStereoscope, SpatialStereoscope
scvi.settings.seed = 0
print("Last run with scvi-tools version:", scvi.__version__)
Last run with scvi-tools version: 1.1.0
Note
You can modify save_dir
below to change where the data files for this tutorial are saved.
sc.set_figure_params(figsize=(6, 6), frameon=False)
sns.set_theme()
torch.set_float32_matmul_precision("high")
save_dir = tempfile.TemporaryDirectory()
%config InlineBackend.print_figure_kwargs={"facecolor": "w"}
%config InlineBackend.figure_format="retina"
Download single-cell data#
Read in expression data. This is a subset of the data you want to map. Here I use a balanced subset of cells from the left ventricle (~ 50K). You can create your own subset according to what you are interested in.
adata_path = os.path.join(save_dir.name, "adata.h5ad")
sc_adata = sc.read(
adata_path,
backup_url="https://ndownloader.figshare.com/files/26153564",
)
sc_adata
AnnData object with n_obs × n_vars = 35928 × 33538
obs: 'age_group', 'cell_source', 'cell_type', 'donor', 'gender', 'n_counts', 'n_genes', 'percent_mito', 'percent_ribo', 'region', 'sample', 'scrublet_score', 'version', 'cell_states'
var: 'gene_ids-Harvard-Nuclei', 'feature_types-Harvard-Nuclei', 'gene_ids-Sanger-Nuclei', 'feature_types-Sanger-Nuclei', 'gene_ids-Sanger-Cells', 'feature_types-Sanger-Cells', 'gene_ids-Sanger-CD45', 'feature_types-Sanger-CD45'
obsm: 'X_pca', 'X_umap'
Preprocess single-cell data#
sc.pp.filter_genes(sc_adata, min_counts=10)
sc_adata
AnnData object with n_obs × n_vars = 35928 × 25145
obs: 'age_group', 'cell_source', 'cell_type', 'donor', 'gender', 'n_counts', 'n_genes', 'percent_mito', 'percent_ribo', 'region', 'sample', 'scrublet_score', 'version', 'cell_states'
var: 'gene_ids-Harvard-Nuclei', 'feature_types-Harvard-Nuclei', 'gene_ids-Sanger-Nuclei', 'feature_types-Sanger-Nuclei', 'gene_ids-Sanger-Cells', 'feature_types-Sanger-Cells', 'gene_ids-Sanger-CD45', 'feature_types-Sanger-CD45', 'n_counts'
obsm: 'X_pca', 'X_umap'
sc_adata.obs["combined"] = [
sc_adata.obs.loc[i, "cell_source"] + sc_adata.obs.loc[i, "donor"]
for i in sc_adata.obs_names
]
sc_adata
AnnData object with n_obs × n_vars = 35928 × 25145
obs: 'age_group', 'cell_source', 'cell_type', 'donor', 'gender', 'n_counts', 'n_genes', 'percent_mito', 'percent_ribo', 'region', 'sample', 'scrublet_score', 'version', 'cell_states', 'combined'
var: 'gene_ids-Harvard-Nuclei', 'feature_types-Harvard-Nuclei', 'gene_ids-Sanger-Nuclei', 'feature_types-Sanger-Nuclei', 'gene_ids-Sanger-Cells', 'feature_types-Sanger-Cells', 'gene_ids-Sanger-CD45', 'feature_types-Sanger-CD45', 'n_counts'
obsm: 'X_pca', 'X_umap'
Remove mitochondrial genes
non_mito_genes_list = [
name for name in sc_adata.var_names if not name.startswith("MT-")
]
sc_adata = sc_adata[:, non_mito_genes_list]
sc_adata
View of AnnData object with n_obs × n_vars = 35928 × 25132
obs: 'age_group', 'cell_source', 'cell_type', 'donor', 'gender', 'n_counts', 'n_genes', 'percent_mito', 'percent_ribo', 'region', 'sample', 'scrublet_score', 'version', 'cell_states', 'combined'
var: 'gene_ids-Harvard-Nuclei', 'feature_types-Harvard-Nuclei', 'gene_ids-Sanger-Nuclei', 'feature_types-Sanger-Nuclei', 'gene_ids-Sanger-Cells', 'feature_types-Sanger-Cells', 'gene_ids-Sanger-CD45', 'feature_types-Sanger-CD45', 'n_counts'
obsm: 'X_pca', 'X_umap'
Normalize data on a different layer, because Stereoscope works with raw counts. We did not see better results by using all the genes, so for computational purposed we cut here to 7,000 genes.
sc_adata.layers["counts"] = sc_adata.X.copy()
sc.pp.normalize_total(sc_adata, target_sum=1e5)
sc.pp.log1p(sc_adata)
sc_adata.raw = sc_adata
sc.pp.highly_variable_genes(
sc_adata,
n_top_genes=7000,
subset=True,
layer="counts",
flavor="seurat_v3",
batch_key="combined",
span=1,
)
Examine the cell type labels
sc_adata.obs["cell_states"].value_counts()
cell_states
EC5_art 2000
PC3_str 2000
EC2_cap 2000
EC1_cap 2000
vCM1 2000
FB1 2000
SMC1_basic 2000
PC1_vent 2000
vCM2 2000
vCM3 2000
vCM4 2000
FB4 1912
EC3_cap 1712
EC6_ven 1292
DOCK4+MØ1 970
EC4_immune 751
CD4+T_cytox 642
NC1 524
Mast 453
FB3 436
CD8+T_cytox 429
LYVE1+MØ1 394
LYVE1+MØ2 390
CD8+T_tem 368
SMC2_art 323
NK 322
FB2 308
FB5 245
vCM5 235
CD16+Mo 234
Mo_pi 227
NKT 224
LYVE1+MØ3 223
CD4+T_tem 170
DC 143
CD14+Mo 132
Adip1 126
MØ_AgP 116
B_cells 112
NC2 102
DOCK4+MØ2 99
EC8_ln 90
NC4 59
MØ_mod 48
NC3 39
Meso 30
Adip2 15
Adip4 11
Adip3 8
NØ 7
IL17RA+Mo 3
NC5 2
NC6 2
Name: count, dtype: int64
Read in visium data#
st_adata = sc.datasets.visium_sge(sample_id="V1_Human_Heart")
st_adata.var_names_make_unique()
st_adata.var["mt"] = st_adata.var_names.str.startswith("MT-")
sc.pp.calculate_qc_metrics(st_adata, qc_vars=["mt"], inplace=True)
st_adata
AnnData object with n_obs × n_vars = 4247 × 36601
obs: 'in_tissue', 'array_row', 'array_col', 'n_genes_by_counts', 'log1p_n_genes_by_counts', 'total_counts', 'log1p_total_counts', 'pct_counts_in_top_50_genes', 'pct_counts_in_top_100_genes', 'pct_counts_in_top_200_genes', 'pct_counts_in_top_500_genes', 'total_counts_mt', 'log1p_total_counts_mt', 'pct_counts_mt'
var: 'gene_ids', 'feature_types', 'genome', 'mt', 'n_cells_by_counts', 'mean_counts', 'log1p_mean_counts', 'pct_dropout_by_counts', 'total_counts', 'log1p_total_counts'
uns: 'spatial'
obsm: 'spatial'
Clean up data based on QC values
fig, axs = plt.subplots(1, 4, figsize=(10, 2))
sns.distplot(st_adata.obs["total_counts"], kde=False, ax=axs[0])
sns.distplot(
st_adata.obs["total_counts"][st_adata.obs["total_counts"] < 20000],
kde=False,
bins=60,
ax=axs[1],
)
sns.distplot(st_adata.obs["n_genes_by_counts"], kde=False, bins=60, ax=axs[2])
sns.distplot(
st_adata.obs["n_genes_by_counts"][st_adata.obs["n_genes_by_counts"] < 1000],
kde=False,
bins=60,
ax=axs[3],
)
plt.tight_layout()
plt.show()
sc.pp.filter_cells(st_adata, min_counts=500)
sc.pp.filter_cells(st_adata, min_genes=500)
sc.pl.violin(
st_adata,
["n_genes_by_counts", "total_counts", "pct_counts_mt"],
jitter=0.25,
multi_panel=True,
)
st_adata
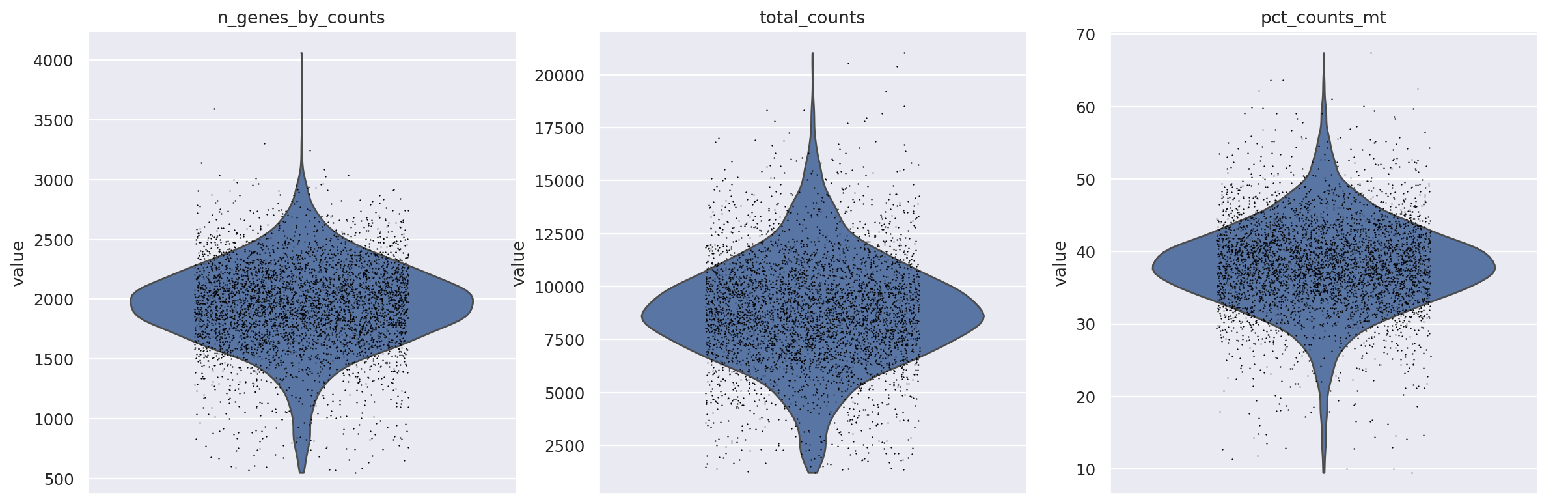
AnnData object with n_obs × n_vars = 4218 × 36601
obs: 'in_tissue', 'array_row', 'array_col', 'n_genes_by_counts', 'log1p_n_genes_by_counts', 'total_counts', 'log1p_total_counts', 'pct_counts_in_top_50_genes', 'pct_counts_in_top_100_genes', 'pct_counts_in_top_200_genes', 'pct_counts_in_top_500_genes', 'total_counts_mt', 'log1p_total_counts_mt', 'pct_counts_mt', 'n_counts', 'n_genes'
var: 'gene_ids', 'feature_types', 'genome', 'mt', 'n_cells_by_counts', 'mean_counts', 'log1p_mean_counts', 'pct_dropout_by_counts', 'total_counts', 'log1p_total_counts'
uns: 'spatial'
obsm: 'spatial'
Learn cell-type specific gene expression from scRNA-seq data#
Filter genes to be the same on the spatial data
intersect = np.intersect1d(sc_adata.var_names, st_adata.var_names)
st_adata = st_adata[:, intersect].copy()
sc_adata = sc_adata[:, intersect].copy()
Setup the AnnData object
RNAStereoscope.setup_anndata(sc_adata, layer="counts", labels_key="cell_states")
Train the scRNA-Seq model
sc_model_path = os.path.join(save_dir.name, "sc_model")
sc_model = RNAStereoscope(sc_adata)
sc_model.train(max_epochs=100)
sc_model.history["elbo_train"][10:].plot()
sc_model.save(sc_model_path, overwrite=True)
Infer proportion for spatial data#
st_adata.layers["counts"] = st_adata.X.copy()
SpatialStereoscope.setup_anndata(st_adata, layer="counts")
Train Visium model
spatial_model_path = os.path.join(save_dir.name, "spatial_model")
spatial_model = SpatialStereoscope.from_rna_model(st_adata, sc_model)
spatial_model.train(max_epochs=2000)
spatial_model.history["elbo_train"][10:].plot()
spatial_model.save(spatial_model_path, overwrite=True)
Deconvolution results#
st_adata.obsm["deconvolution"] = spatial_model.get_proportions()
# also copy as single field in the anndata for visualization
for ct in st_adata.obsm["deconvolution"].columns:
st_adata.obs[ct] = st_adata.obsm["deconvolution"][ct]
Visualise populations#
Et voilá, we have now an anndata object that contains the inferred proportions on each Visium spot for each cell type in our single cell reference dataset.
In this example we can observe how nicely the arterial endotehlial cells (EC5_art) and the venous endothelial cells (EC6_ven) are highlighted in the areas were we expect to see cardiac vessels based on the histology of the sample.